Google announced the release of RT-2, a vision-language-action (VLA) model. It is a Transformer-based model trained on text and images from the web, allowing it to directly output robotic actions. Just as language models learn general ideas and concepts from web text, RT-2 gains knowledge from web data to affect robot behavior.
Challenges in Real-World Robot Learning
Creating helpful robots capable of performing general tasks in the real world has always been challenging. Unlike chatbots, robots require “grounding” in the physical world and must possess a deep understanding of their abilities. Training a robot is not just about learning facts about objects like apples; it involves recognizing an apple in context, differentiating it from other objects like red balls, comprehending its appearance, and most importantly, knowing how to pick it up.
Historically, achieving this level of competence has demanded training robots on billions of data points, covering every conceivable object, environment, task, and situation in the physical world. This process has been time-consuming and costly, making it impractical for most innovators.
The RT-2 Approach to Learning
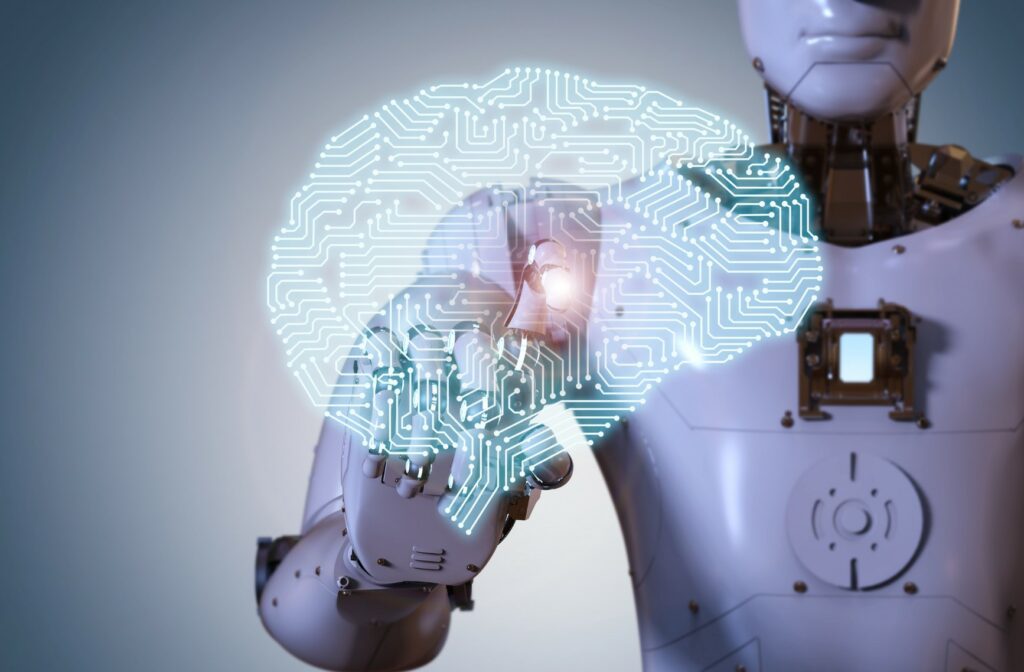
Recent advancements in AI have improved robots’ reasoning abilities, enabling them to handle multi-step problems through chain-of-thought prompting. Vision models like PaLM-E have helped robots better understand their surroundings. RT-1 demonstrated that Transformers, known for their ability to generalize information across systems, could facilitate cross-robot learning.
However, robots relied on complex stacks of systems, with high-level reasoning and low-level manipulation systems communicating with each other imperfectly. This process resembled a game of telephone – a high-level instruction had to be conveyed to lower-level systems to execute actions. RT-2 reportedly transforms this approach by consolidating all functions into a single model. Not only can it perform complex reasoning like foundation models, but it can also directly output robot actions. RT-2 shows that with a small amount of robot training data, it can transfer the concepts embedded in its language and vision training data to direct robot actions, even for tasks it has never been trained to do.
For instance, previous systems required explicit training to identify and pick up trash before learning how to throw it away. However, RT-2, having absorbed knowledge from a large corpus of web data, can already recognize trash without explicit training and even knows how to dispose of it, despite never being specifically taught that action. Additionally, RT-2 reportedly can comprehend the abstract nature of trash, recognizing that what was once a bag of chips or a banana peel becomes trash after being consumed. This level of understanding stems from its vision-language training data, allowing it to accomplish tasks efficiently.
RT-2 Outperforms RT-1 by 30% in “Unseen” Tasks
RT-2’s ability to transfer knowledge into actions offers the potential for robots to rapidly adapt to new situations and environments. In tests involving over 6,000 robotic trials, RT-2 performed as well as its predecessor, RT-1, on tasks from its training data, known as “seen” tasks. However, it significantly outperformed RT-1 on novel, unseen scenarios, achieving a success rate of 62% compared to RT-1’s 32%.